Near Quantum
Our Near Quantum Platform
Our Near Quantum Solutions use our proprietary algorithms, QUBO’s and specialized digital optimization software and methods along with HPC hardware to deliver high quality Quantum type results. Based on our R&D on medium scale size problems, we have been able to successfully demonstrate comparable results versus a hybrid quantum model. The platform is scalable to handle larger problems.
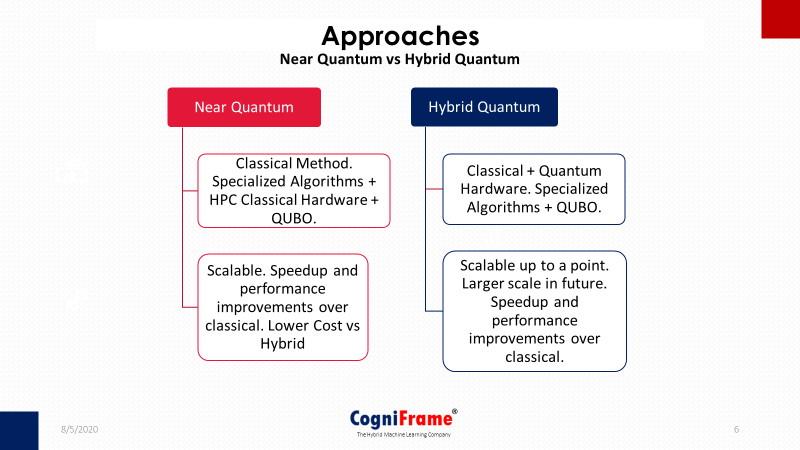
The combination of Near Quantum and Hybrid Quantum provides a path to a dual strategy; problems with a very large number of features/variables can be optimized using Quantum while smaller or mid sized ones can be handled using our high performance classical model with superior results vs existing traditional methods. Also, areas that involve constant optimization would benefit from the lower cost Near Quantum approach. The process involved in both scenarios involves creation of QUBO's. But there are some differences in the overall construct and tuning process. The Near Quantum platform is scalable and can be accessed via simple API’s.
At CogniFrame, we believe that in the long run Near Quantum approaches will work side by side Hybrid Quantum approaches as this provides the best financial business case outcome.
Our Solutions
Feature Selection
CogniFrame’s OFS Algorithm helps helps solve what is traditionally considered an NP-Hard problem (due to high dimensionality) and It helps reduce features of the data without losing on quality of outcome leading to improved Machine Learning Training Time. It also helps uncover mutual information between weaker features that cannot be uncovered using traditional approaches and existing algorithms optimally.
Read MoreAnonymization
While it is beneficial for organizations to share data with each other, a key concern is protecting the sensitive information without sacrificing the quality of information. The problem then become transforming a high-sensitive dataset into a privacy-preserving, low risk data which can be safely shared with any entities ranging from researching teams to business partners. CogniFrame’s anonymization algorithm ensures that the identify of an individual is non-distinguishable among other records, providing an optimal anonymization method without sacrificing the information in the data.
Read MoreClustering
There are inherent problems with clustering because it is a NP-Hard problem with High Dimensionality. This is because as you increase the number of unlabelled examples, clustering becomes too complex for classical methods. Many traditional clustering techniques do not provide the optimal solution for data mining scenarios. CogniFrame’s near quantum solution can solve this NP-hard problem, finding the optimal clusters for client needs.
Read More